INFLATION COOLS BUT LESS THAN EXPECTED
The June read in the Consumer Price Index (CPI) supported the position that inflation is receding. US inflation came in lighter than expected rising 0.1% month over month (through May month end) which was in line with economist’s estimates.
The year over year number fell for the eleventh consecutive month. Based on May’s month end data, the year over year CPI came in at 4.0% versus 4.9% for April well down from last year’s 9.1% peak number.
The core CPI – which strips out volatile items like food and energy costs – was very much in line with expectations, rising 0.4% in May versus 0.4% in April and forecasts for 0.4%. Core CPI slowed to 5.3% year over year which was in line with expectations and down from 5.5% in April.
What the numbers are telling us – the same story is unfolding in Canada – is that the cost of accommodation and services are holding sway on the numbers. Increases in shelter costs and used vehicles pushed the index higher which was offset somewhat by declines in prices for fuel oil, new vehicles, and groceries.
Shelter accounts for approximately one third of the CPI basket and because of its influence on the month over month data, it is helpful to understand how it is calculated. Shelter costs are not, as many believe, a reflection of the absolute price increase in median home price across the country. Rather, shelter inflation is calculated using a measure known as “owner’s equivalent rent,” which attempts to measure what a given house would be able to collect if rented. Using this metric addresses the fact that shelter costs for homeowners and renters can vary substantially. The owner’s equivalent rent aims to smooth out the attendant distortions.
Tamping down shelter inflation is a two-step process. Since rents tend to lag, residential property values must first recede. Theoretically, as property values retreat future rent increases will moderate. At least that’s the theory.
The problem is lack of supply has caused an escalation in residential property values. Compounding the problem is the attendant cost of carry associated with higher mortgage rates. As central banks raise rates to slow economic activity, they are inadvertently escalating the major component in the CPI which means that getting inflation from where it is to target will be a formidable challenge.
Given the challenges surrounding shelter costs, it should not have come as a surprise that the US Federal Reserve (Fed) decided to hold rates steady at the June FOMC meeting. This was not a pause or pivot, but a so-called “skip” strategy. Think of the June decision as a conditional pause much like we saw with the Bank of Canada (BOC) that moved from the sidelines by raising their benchmark rate by ¼% at the June meeting. The BOC had been on a rate hike hiatus since January.
The Fed’s objective is to evaluate the impact of hikes that have already occurred. Depending on how the economy responds, will determine whether another rate hike is likely at the July meeting.
There are subtleties underpinning a “skip” strategy. So many in fact, that it makes one wonder if descending into a nuanced rabbit hole is worth the effort. Fair question!
From our perspective, it is worth the effort because of the impact nuanced decisions have on market perception. Three months ago, investors would have viewed a decision not to raise rates as a sign that the Fed was finished and there would be a period of stability that would lead to rate cuts by year end.
The decision to skip a rate hike implies that more hikes are on the table. In our view the committee will likely re-engage with another rate hike at the July meeting depending on incoming data. Most importantly, we think there is virtually no chance that the Fed will cut rates in 2023.
In terms of the data, the main concern will be whether shelter costs become entrenched. That’s not the prevailing view. The cost of home ownership is highly dependent on mortgage rates which by all accounts are close to peaking. The costs associated with renting are more complex. Low unemployment, demographics, demand, and mortgage costs directly impact what a landlord can charge. No quick fixes among these factors.
Beyond housing data, the Fed has been taking a closer look at the “super-core” inflation rate. An obscure dataset that excludes food, energy, and shelter, focusing instead on raw material costs and services such as those provided by lawyers, plumbers, gardeners, and hairdressers that remain well above trend.
Historically the Fed had focused on “core” inflation because the components tended to be less volatile and by extension, transitory. The super core concept gained notoriety after being outed by Chairman Powell and Nobel laureate economist Paul Krugman. In Powell’s view, removing components that cause inflation spikes and focusing on elements with entrenched price behavior provides a better measure of the longer-term direction of prices across the broader economy.
The Fed spends a lot of time drilling down into the super core components with specific emphasis on labor costs within the service sector. For instance, prices of haircuts and personal grooming services rose from January to December 2022 from a year ago, according to a Wall Street Journal analysis. In comparison, prices of televisions fell in the same period, highlighting that the issue with persistent inflation could be due to the rising prices of services rather than goods.
The cost of services is the direct result of a tight labor market that has been more robust than anyone expected. That said, some cracks seem to be emerging. In Canada, for example, the May labour report showed a decline of 17,000 jobs (note this report was released after the aforementioned ¼ point rate hike) which is the first decline after eight consecutive months of job growth.
A more muted story in the US. Unemployment is ticking up but a rate of 3.7% is not likely to moderate Fed policy. More importantly is the surprising resilience in the US economy. It’s a glass half full or half empty depending on one’s positioning.
The half full perspective implies that a robust economy raises the likelihood that the Fed can orchestrate a soft landing. The half empty line of reasoning suggests further rate hikes will impinge profits and delay any return to normalcy.
Then there is the super core focus underpinning Fed decisions. The Fed has already raised interest rates eight times since early 2022 pushing up the cost of borrowing which theoretically should encourage people to save. That’s a tough sell to American consumers who are typically aggressive spenders.
THE RETURN OF THE BULL?
The longest bear market in 75 years is over. At least according to the bull bear thresholds that signal sentiment shifts. On June 8th, 2023, the S&P 500 composite index closed above 4,292 (4,293.93 to be exact) which marked a 20% rally from the October 12th, 2022, closing low of 3,577.
Stocks have been supported by a resilient economy, better than expected first quarter earnings, a surprisingly robust jobs market and the prevailing view that the aggressive rate hiking campaign is coming to an end.
Having said that, the rally has been narrowly focused. Most of the year-to-date performance can be attributed to the “Magnificent Seven” which are seven of the biggest technology names including Nvidia, Microsoft, Meta Platforms, Alphabet (i.e., Google), Apple, Amazon and Netflix.
If we are to see further gains, a couple of things will need to happen; 1) the economy will need to trudge along without slipping into a serious recession (a mild recession would be okay) and 2) the rally will have to broaden out (which it seems to be as of late).
It is hard to imagine stocks that are trading at 60 times forward earnings will continue to explode to the upside. Although the FOMO (Fear Of Missing Out) trade has taken hold among these companies now flying high on the wings of generative A.I., which many tech analysts believe will be transformative.
It will also require analysts to reassess their positions. At some point they will have to wake up to the idea that 2023 may not be as bad as originally forecast as recession expectations are getting pushed out to 2024. Even then, the recession may be milder than anticipated.
None of this is a foregone conclusion. However, there is evidence that when markets rally 20% from their 52-week low, further gains are likely. Especially if the S&P 500 index can cement its position above the 4,292-demarcation line.
Historically, since 1950 there have been 13 occasions where equity markets rallied 20% from the 52-week lows and 10 of those times led to average gains of 17.7% in the ensuing twelve months. It didn’t work when equity markets were at their worst inflection points propelled by irrational exuberance. Think of the tech boom bust in 2000 where there were two false starts and the great financial crisis of 2008 where things went from bad to worse.
We do not see irrational exuberance in the current market as many investors are not positioned for a bull rally in stocks. According to analysts the short interest (bearish positions) is higher than it was during the great financial crisis and for those investors to re-position, they would have to close out their shorts and purchase shares in companies they want to hold.
Then there is the horde of retail investors holding funds in money market accounts. Estimates suggest this stockpile could be greater than US $1 trillion. If those investors start moving from the sidelines it will provide serious oomph that would extend the current upward bias.
Another consideration is the CBOE Volatility index (symbol VIX, see chart below) which is at a three-year low. That tells us that the excess volatility experienced in 2022 has given way to a low volatility regime more in line with prior bull markets seen from 2014 through 2018.
Goldman Sachs, one of the most influential Wall Street firms, has also jumped on the bull bandwagon by recently upping their year-end target for the S&P 500 index from 4.000 to 4,500. Goldman cited improved prospects for a soft landing and an upbeat earnings trajectory as reasons for the revised forecast.
Goldman view is that the probability of a U.S. recession in the next twelve months has declined from 35% to 25%. The downward revision was supported by a couple of factors; 1) the debt ceiling dispute was resolved which will result in minor spending cuts that are expected to have a minimal impact on the economy and 2), stress in the banking system has all but abated, although turbulence among regional banks will likely shave 0.4% from real (inflation adjusted) GDP in 2023. Goldman believes the US GDP will rise by 1.8% in 2023 which is well above expectations among private economists and the Fed.
The S&P 500 trades at about 19 times current earnings. By historical standards the market is not cheap. However, as mentioned, the above average price to earnings multiple within a capitalization weighted index is the result of outsized gains in some of the largest constituents (the Magnificent Seven).
Historically, when the market experiences sharply narrow breadth it has been followed, according to Goldman, by a “catch-up from a broader valuation re-rating.”
Whether that comes to pass depends on how investors weigh the risks of continued Fed tightening that may cause a severe prolonged recession (see Fat Tail Risk), versus the prospects of improved efficiencies propelled by generative A.I. and the positive impact that it could have on corporate profit margins.
FAT TAIL RISK
The promised efficiencies associated with generative A.I. may not be enough to offset macro-economic factors that, according to the Conference Board, will impinge on global growth. Persistent inflation, Fed hawkishness, a slowdown in bank lending as regional banks reset their lending terms, reduced government spending mandated as part of the US debt ceiling negotiations, weakness in personal consumption patterns and business investment will cause GDP growth to slow to 1.0% (the base case for most economists) in 2023 and then fall to 0.0% in 2024.
The largest recession risk is consumer spending patterns which have fallen in four of the last six months. What we are seeing is that consumers appear willing to spend on services but not so much on goods. Most likely spending on goods will continue to abate under the weight of higher interest rates.
As for services, we did get some positive feedback (at least positive in terms of how it might impact further rate hikes) from the latest PMI data that seems to suggest that the service sector is beginning to cool. The best guess among economists is that consumer spending will grow through the summer but will begin to contract late in the third quarter of 2023.
Consumer spending has been propped up by a couple of factors. The first is excess savings associated with government programs designed to mitigate pandemic related stresses. However, according to the Conference Board, those excess savings are being depleted and will probably run out by the end of the year.
The second is the robust labour market that has resulted in outsized wage gains. However, unemployment is ticking up and over the next quarter should moderate the tight labour market. That should dampen wage demands through the remainder of 2023. One other factor that will have a direct impact on millennial spending is the reintroduction of student loan payments which will begin in September.
Business investment remains weak and will likely worsen under the weight of higher interest rates and tight loan requirements. More specifically, business investment is not just about higher rates but the trajectory of rates. On that front, we do not envision any rate cuts until sometime in 2024.
A similar story is playing out in residential investment which is particularly sensitive to Fed policy. This segment has already contracted and will likely continue to do so until we see rate cuts which again, are not expected until 2024.
Even government spending, which was one of the few growth drivers of the economy, should recede. Notes the Conference Board, “federal non-defense spending benefited from outlays associated with infrastructure investment legislation passed in 2021 and 2022. However, reductions in discretionary outlays (US $1.5 Trillion over 10 years) detailed in the Fiscal Responsibility Act (which averted the debt ceiling crisis) will limit overall government spending and act as a drag on growth later this year and early next.”
Of course, many of the issues associated with recession risk hinge on the trajectory of inflation. While we applaud the positives associated with CPI contraction over the past eleven months, as noted in our earlier discussion (see: Inflation Cools), getting from 4% inflation to target will take some time. The prevailing forecasts suggest that year-over-year inflation will fall to 3% by year end and if we are lucky, get to target by the fourth quarter of 2024. That base case should support current equity valuations. If the data comes in better than expected, that will probably light a fire under the “catch-up” trade.
GENERATIVE ARTIFICIAL INTELLIGENCE
There has been much talk about Generative Artificial Intelligence (A.I.) in terms of the potential benefits (improved efficiencies and profit margins) and the inherent risks (end of humankind). The truth is probably somewhere in the middle.
While it is not possible to do anything but scratch the surface on this topic, we believe it is important for investors to have a rudimentary understanding of the benefits and pitfalls and perhaps more importantly, how that will effect profit margins in various industries.
Background:
A.I. is not new. It has been around for years and has some bearing in our daily lives. Your Apple iMessage service provides word prompts when typing a text message. Word processors self-correct mis-spelled text, editor functions analyze grammar and writing style. On a grander scale: self-driving cars, robotic assembly lines, MRNA vaccines, logistical support systems… etc.
So… if we have been tinkering with A.I. since the turn of the century, why all the hype? The difference is that most of the systems we have been using are what is known as traditional A.I. These applications rely on specific rules that generate pre-defined responses. Think of the robots in an automobile manufacturing plant that perform a single redundant function as vehicles move along the assembly line.
What makes generative A.I. different is that it utilizes algorithms and models capable of generating original content with a degree of creativity. That latter point is why it is becoming influential in the Arts: namely in the production of original music, literature, and design.
Before getting into the investment thesis, allow me some latitude to explain how generative A.I. works. That said, I lack formative training in computer programming which limits any ability that I might have to explain the nuances of generative A.I. As such, I will step to the sidelines and let Chat GPT (GPT text in italics) explain the building blocks of generative A.I.
Understanding Generative A.I.
Generative A.I. can be broadly defined as a subset of machine learning that focuses on the creation of new data based on patterns and examples from existing datasets. Unlike traditional A.I. models that rely on explicit rules and pre-defined responses, generative A.I. systems can generate novel outputs that resemble the original data they were trained on. These systems leverage deep learning techniques, such as generative adversarial networks (GANs), variational autoencoders (VAEs), and recurrent neural networks (RNNs), to learn complex patterns and generate realistic content.
Generative Adversarial Networks (GANs)
One of the most prominent techniques used in generative A.I. is the Generative Adversarial Network (GAN). GANs consist of two interconnected neural networks: a generator and a discriminator. The generator attempts to create realistic outputs, such as images or text, while the discriminator aims to distinguish between real and generated content. Through an iterative process of competition and collaboration, GANs refine the generator’s abilities to produce increasingly convincing outputs. This adversarial training leads to the generation of high-quality, original content that exhibits creative elements.
Variational Autoencoders (VAEs)
Variational Autoencoders (VAEs) represent another powerful approach to generative A.I. VAEs are probabilistic models that aim to learn and encode the underlying structure of a dataset in a lower-dimensional latent space. By sampling from this latent space, VAEs can generate new data points that resemble the original distribution. VAEs offer a structured and controllable way to generate content, allowing users to explore and manipulate various attributes of the generated outputs. This flexibility makes VAEs well-suited for applications in creative domains, such as image synthesis, music composition, and text generation.
Applications of Generative A.I.
As you can imagine generative A.I. is being embedded in a broad swath of industries. We have already witnessed the creation of original artwork, music scores, and editorial content. In its purest form, it can generate ideas for artists, play basic rhythms and harmonies that can provide a foundation for new musical scores and background sounds for video games.
Chat GPT has demonstrated its impact on literature. As generative A.I. sifts through limitless data available on the Internet it is learning to generate coherent responses, with contextually appropriate sentences that end with appropriate conclusions. Again, in its purest form, it can help authors overcome writers block, provide inspiration and drafts that can be refined and expanded upon. But, as you can imagine, there are challenges that have raised concerns among generative A.I. developers.
Implications and Challenges
The potential applications for generative A.I. are limitless. However, the rise of generative A.I. brings both excitement and concerns. On one hand, it opens new horizons for creativity, collaboration, and human-machine interaction. On the other hand, it raises ethical questions and challenges. For instance, there are concerns about intellectual property rights when generative A.I. is used to create art or music. Additionally, the potential misuse of generative A.I. for malicious purposes, such as generating deepfake videos or spreading misinformation, poses significant challenges for society.
Moreover, generative A.I. models are not immune to biases and can inadvertently perpetuate or amplify existing societal biases present in the training data. Careful consideration must be given to data selection, preprocessing, and model training to mitigate these biases and ensure fairness and inclusivity in the generated outputs.
Misuse and Malicious Activities
Generative A.I. can be misused for various malicious purposes. For example, it can be used to create highly convincing deepfake videos, spreading misinformation or defaming individuals. This raises concerns about identity theft, fraud, and the erosion of trust in media and information sources.
Intellectual Property and Copyright Infringement
Generative A.I. models trained on existing copyrighted works can inadvertently generate outputs that infringe upon intellectual property rights. This raises legal and ethical questions regarding ownership, attribution, and fair use of generated content. Resolving these issues is crucial to ensure that generative A.I. respects and upholds intellectual property laws.
Amplification of Biases
Generative A.I. models are trained on large datasets, which may contain inherent biases present in the data. These biases can be inadvertently perpetuated or even amplified in the generated outputs. For instance, if a generative A.I. model is trained on biased text data, it may generate biased or discriminatory text. Addressing and mitigating these biases is essential to ensure fairness and inclusivity in generative A.I. applications.
Ethical Implications
Generative A.I. raises ethical concerns, particularly when it comes to creating content that imitates human creativity. For instance, when AI-generated content is presented as the work of human artists or authors, it can raise questions about authenticity, authorship, and the value attributed to human creativity. Clear guidelines and transparency are necessary to avoid misleading or deceiving consumers.
Security and Privacy Concerns
The generation of realistic content by generative A.I. models can be exploited to deceive users and gain unauthorized access to systems. For instance, voice or image synthesis can be used for impersonation or to bypass security measures. Ensuring robust security measures and user authentication protocols is crucial to prevent misuse of generative AI technology.
Unintended Consequences and Unforeseen Biases
Generative A.I. models can sometimes produce unexpected or undesirable outputs, even with the best training data. These unintended consequences may have negative implications in real-world applications. For instance, an A.I. language model may generate offensive or harmful text despite efforts to train it responsibly. Continuous monitoring, evaluation, and improvement of generative A.I. models are necessary to minimize such risks.
Dependency and Human Redundancy
As generative A.I. becomes more sophisticated, there is a concern that it may replace or devalue human creativity and expertise in certain domains. This raises questions about job displacement and the need for reskilling or redefining the roles of individuals working in creative fields.
Addressing these risks requires a comprehensive approach involving collaboration among researchers, policymakers, industry stakeholders, and the wider society. It involves establishing clear ethical guidelines, promoting responsible use of generative A.I., fostering transparency and accountability, and continuously updating regulations to keep pace with technological advancements. By proactively addressing these risks, we can harness the benefits of generative A.I. while mitigating potential harm.
The Investment Thesis
What gets interesting from an investment perspective is how the use of generative A.I. can boost profit margins and improve efficiencies. Take the advances in code writing, scientific research and in the future, optimization of trading platforms as examples.
Meta Platforms (formally Facebook) has laid off more than 40,000 employees to improve margins. Many of those caught in the shuffle were engineers (code writers) earning on average US $200,000 annually. That is a major cost saving to Meta if generative A.I. can produce the algorithms used in target marketing. Our best guess is that generative A.I. will not only produce results more cost effectively, but the resulting algorithms will also be more efficient and will improve over time as the machines learn what works best. It’s no wonder that Meta has risen so dramatically since the beginning of the year.
We have seen a similar story with Microsoft that has made a major investment in Open A.I. Generative A.I. is already embedded in Microsoft’s Bing search engine and the scheduled update in M365 (Microsoft Office) that is expected to be released this Fall will incorporate similar components. The other factor that plays into the Microsoft story is their Azure cloud offering. Generative A.I. resides in the cloud and requires significant storage for it to work. That puts it squarely in Microsoft’s wheelhouse.
The other big tech names are already jumping into the fray. Google in terms of its own search engine and Amazon for the benefits derived from improved logistics (i.e., last mile delivery). From our perspective, generative A.I. is a transformative disruptor that will influence every sector of modern economies.
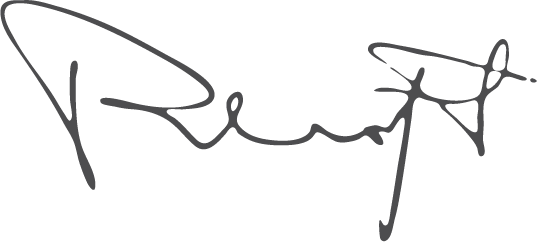
Richard Croft, Chairman & CIO